NIH Chest X-ray Classifier
In my Applied Fundamentals of Machine Learning course (APS360) at UofT, I collaborated with a group to develop neural network models for classifying Chest X-ray Images. Utilizing the NIH Chest X-rays TFRecords dataset, we trained several convolutional neural network models using PyTorch.
To address class imbalance, we curated the dataset to focus on the three most prominent diseases: Atelectasis, Infiltration, and Effusion, while also including one third of the No Finding data. This balanced dataset was crucial for our model training. Although the original data was stored in TensorFlow format, we seamlessly converted it to PyTorch tensors to align with our course curriculum.
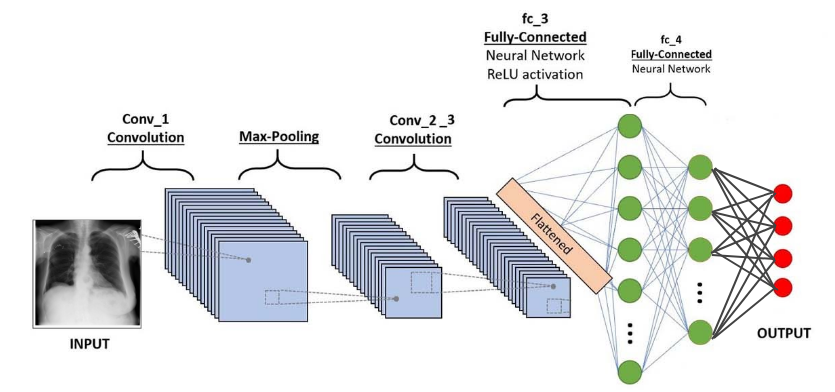
Our final CNN model, illustrated above, comprised three convolution layers with max pooling, followed by a fully connected network. Despite our efforts, we encountered challenges in achieving optimal performance, attaining only a 72% accuracy on our test dataset.
GitHub Project Repository